Streamlining Operations with AI: Michał Zieliński's Journey at Classic Driver
In this conversation, we chat with Michał Zieliński, product manager at Classic Driver, a platform for classic and collectible cars. Michał, who joined the company in January 2024, shares his experience using AI tools like ChatGPT to streamline internal processes, particularly automating meeting notes. He discusses the challenges, lessons learned, and the importance of experimentation and iteration when implementing AI in small teams. Michał’s insights offer valuable guidance for anyone looking to integrate new technologies into their workflow.
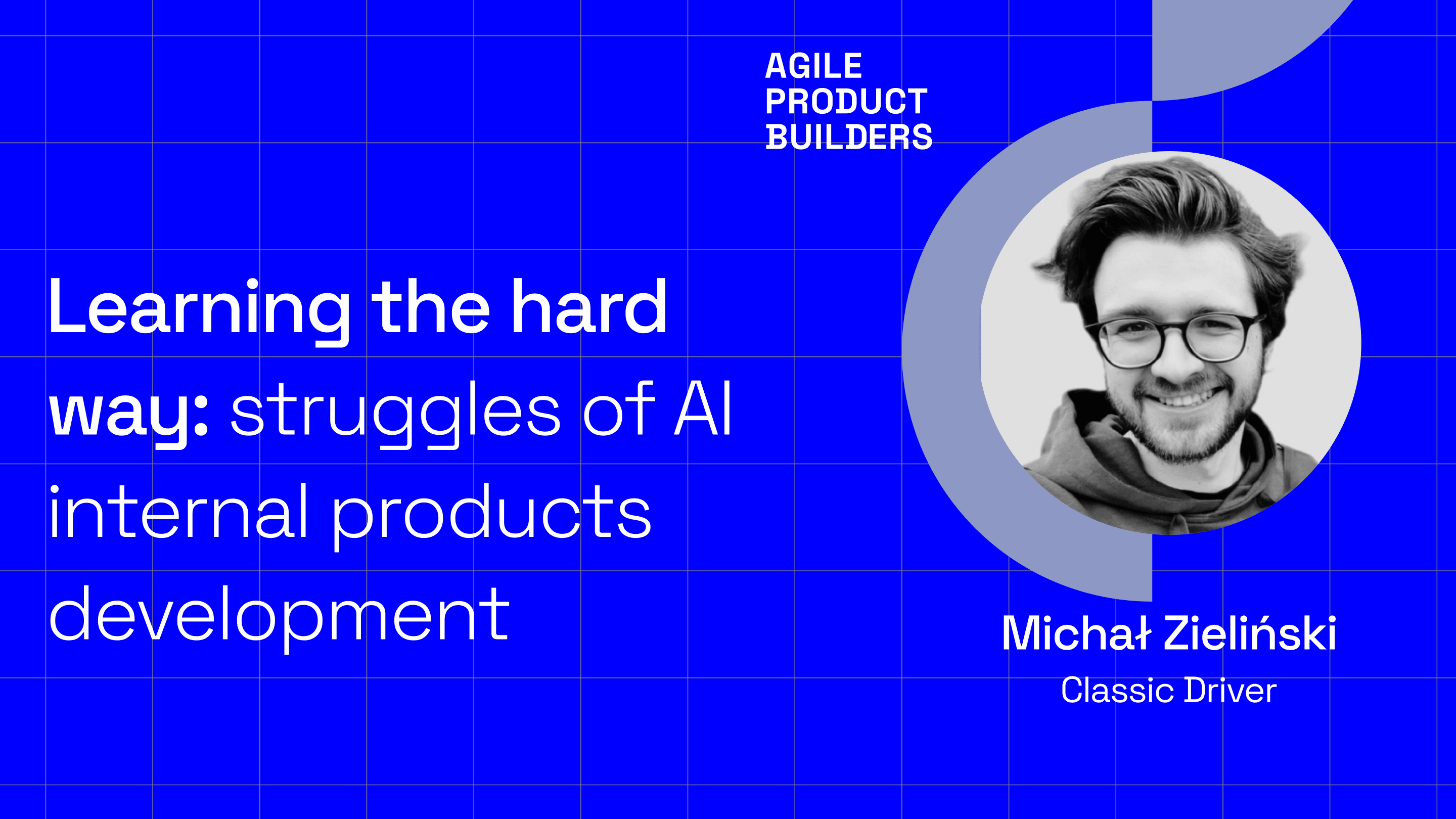
Table of contents
Oskar: Hi Michał, it’s great to chat with you today. You’ve had a chance to experiment with AI tools at Classic Driver, particularly in streamlining internal processes like meeting notes. What prompted you to start using AI tools in your day-to-day work, and what kind of challenges were you hoping to address?
Michał: Hi, Oskar! Thanks for having me. I’m Michał Zieliński, and I’m currently a product manager at Classic Driver. We’re an international platform for buying and selling classic and collectible cars. We also run an online magazine and a small e-commerce store. Our mission is to connect car enthusiasts and create a community around classic cars.
When I joined Classic Driver in January 2024, I came from a much larger company with around 1,500 employees, so the shift to a smaller team of fewer than 20 people was a big change. The smaller team meant things operated a bit differently, and I quickly noticed several operational inefficiencies that could be solved by creating some internal tools. One of the first problems I identified was something as simple as taking meeting notes. It might sound trivial, but it was time-consuming, especially when I had to write them from scratch after every meeting. I started thinking, “Can AI help automate this process?”
Oskar: Before diving into creating your own solution, did you explore existing tools that could transcribe meetings and take notes, or were you set on building something custom, like using OpenAI?
Michał: Initially, I did look into existing tools. There are paid solutions out there, but they were expensive, and our needs were pretty minimal. So, we wanted to explore more cost-effective options. That’s when I decided to dig into technologies like ChatGPT. I wanted to experiment with what the AI could do, learn how it worked, and understand where it might fall short.
Oskar: And what was the process of building the tool like? Did you follow any specific framework or methodology in developing it?
Michał: I wouldn’t say there was a formal framework; it was more about how I think about internal tools. The goal was always to build something that was efficient—quick to implement, not necessarily perfect, but something that would add value right away. This is a great approach for internal tools, especially when time is of the essence.
So, I started with some basic experiments using ChatGPT for meeting transcriptions. But soon, I ran into a problem: the AI started generating what’s called “hallucinations” — incorrect information, like new job titles that didn’t exist. That’s when I realized I needed to take a step back and reassess the approach.
Oskar: That’s an interesting story. How long did it take you to create the first proof of concept (PoC)? What did those early attempts look like?
Michał: It took a few weeks to get something working, but honestly, a lot of that time was spent on trial and error. Initially, I was manually taking notes during meetings and comparing them with what the AI generated to see how close the results were. The key was to set the right context for the AI, so it could generate something useful, but also to understand where it might fail.
I also made sure not to use ChatGPT for anything involving sensitive data. Over time, I found that the AI was good at summarizing meetings, but it needed some fine-tuning to avoid those initial errors. I tried different models to see which one would provide the best results for our needs.
Oskar: How did you assess the effectiveness of the tool? Do you have any metrics that helped you evaluate the success of the project?
Michał: I focused on two main metrics. First, I looked at feedback from the team. After sending out two versions of meeting notes—one written by me and one generated by the AI—I asked them which one they preferred. When no one raised objections, I knew we were on the right track.
The second metric was time efficiency. Before using AI, creating meeting notes would take me up to an hour. Once we automated the process, I could do it in about 5-10 minutes. That was a huge time-saver for me and allowed me to focus on other tasks.
Of course, there were some errors, like missing important details, but overall, the process became much faster.
Oskar: I understand that efficiency was key, but how would you assess the impact of the tool from the perspective of the entire team? Did you notice any improvements in the organization?
Michał: That’s harder to quantify, but we definitely saw some positive effects. For example, team members who couldn’t attend meetings were able to catch up more easily because they had full meeting notes that included all the key decisions and action points. This helped align the entire team. It’s tough to measure exactly, but from an organizational perspective, I think it definitely made the team more connected and on the same page.
Oskar: Looking back, what would you have done differently if you were to start this project again?
Michał: If I knew then what I know now, I would have started experimenting with different models much earlier. At first, I had a general understanding of how these AI tools worked, but I didn’t dive deep into the specifics of which models would be best for different tasks. If I could go back, I would research more thoroughly at the outset to figure out which models could offer the best results for what we needed.
Oskar: Do you have any advice for others who might want to implement AI in their products or internal processes?
Michał: Two key takeaways: First, don’t get discouraged when AI tools don’t work perfectly at first. Early results may not meet your expectations, but that’s part of the learning process. Don’t give up too soon. And second, focus on delivering value quickly. For internal tools, it’s important to iterate and improve over time. Don’t wait for something to be perfect before deploying it—just get something working, then refine it as you go.
Oskar: Those are great tips, Michał. Thanks so much for sharing your experience with us today!
Michał: Thank you, Oskar. It was a pleasure to talk with you!
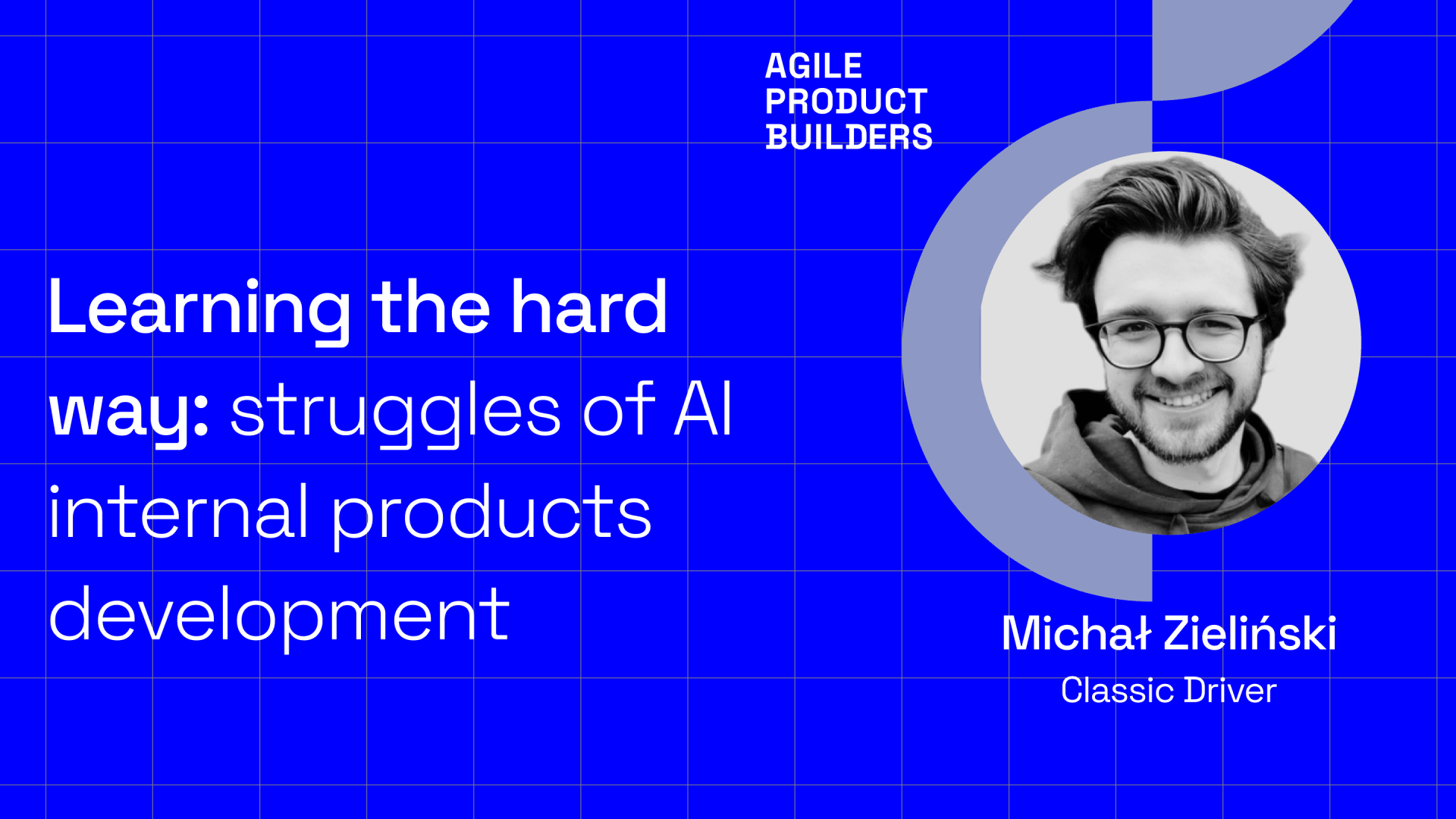
Share this article: