Quantitative Research: Methods, Examples and Best Practices
UX research is a systematic and complex process which is aimed at identifying and studying the needs, struggles, and pain points of the target group. Depending on the research goal that the product team wants to achieve, they can employ different UX research methods tailored to provide them with the most insightful and informative data. These methods typically fall into either the quantitative or qualitative categories of research. The fundamental difference between them is the type of data you require to answer the research question. Read on to learn more!
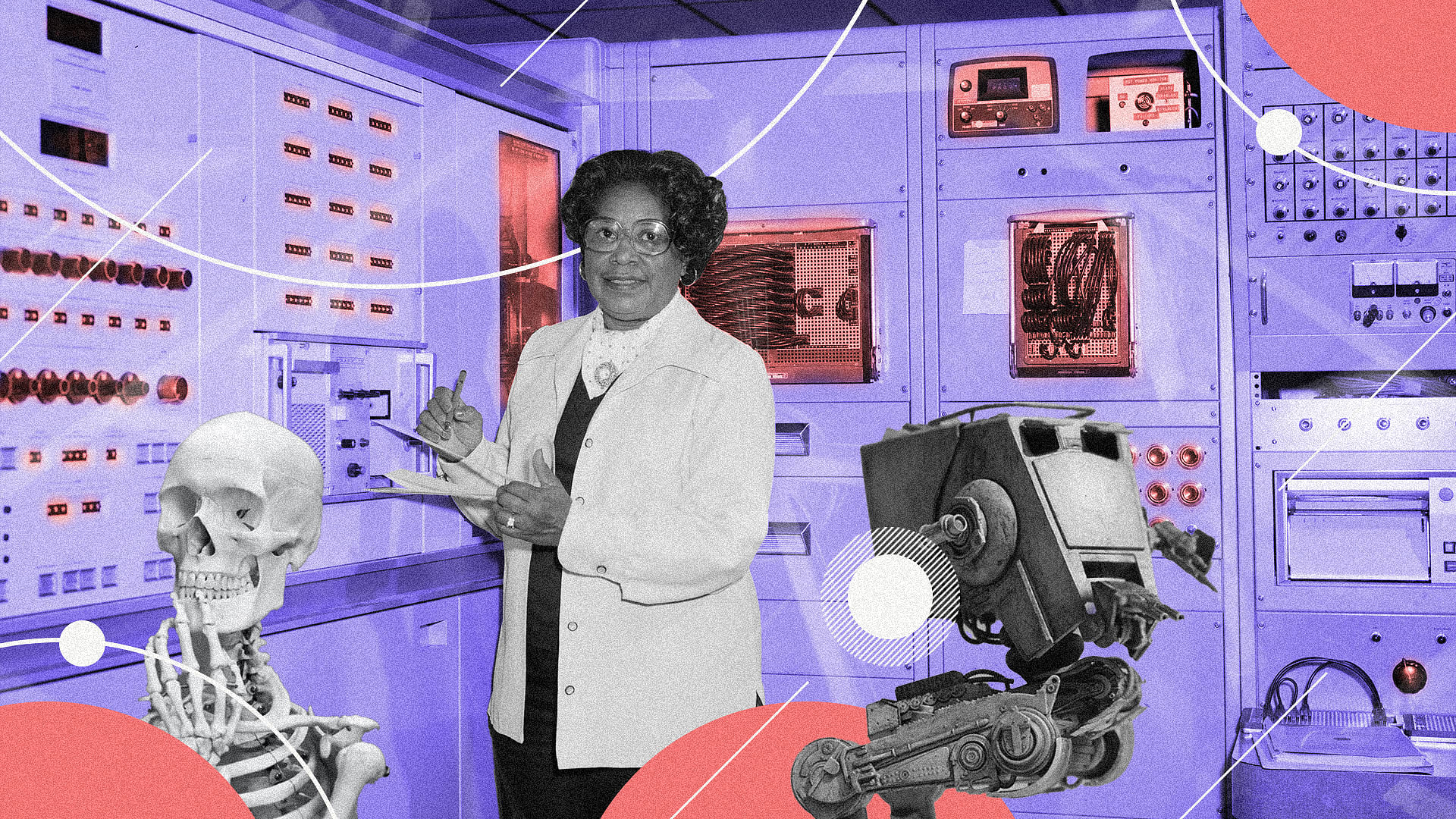
Table of contents
What is the difference between qualitative and quantitative UX research?
Qualitative UX research
Qualitative UX research is focused on users’ behaviors, motivations, and attitudes, and collecting subjective perspectives. In this type of UX research, the researcher typically observes users’ behavior in order to:
- discover problems or opportunities,
- gain some deeper understanding of what matters to our target group and why,
- obtain some insights about people’s behavior in their actual context and environment,
- inform design decisions.
Quantitative UX research
Quantitative UX research is about collecting and analyzing statistical data to identify common patterns in users’ behavior. This methodology allows researchers to:
- statistically validate or invalidate the hypotheses they’ve built based on qualitative data,
- compare how well different design alternatives perform (A/B tests), preference tests),
- analyze users’ behavior at a greater scale in order to spot points of possible opportunity and problems,
- check how well our design decisions work in terms of conversion and ROI.
Basically, qualitative research answers what & why, while quantitative research answers how much & how many, so by using them in combination, we can get the full overview of our users’ attitudes and behaviors and get the most out of our research.
Qualitative and quantitative UX research can be compared, but it’s important to remember that overall neither of these research types is better than the other, and they should not by any means be mutually exclusive. On the contrary, combining statistically significant data with the context and details obtained while observing users is what typically gives the best insights.
Today we’re going to take a closer look at quantitative UX research methods and how to make the most of them.
Quantitative UX research methods with examples
Quantitative UX research provides us with data from indirect sources, such as specific measurements and instruments. In studies using quantitative research methods, the results go through mathematical analysis because the volume of data is so large that it’s reasonable to summarize it with the help of mathematical equations or calculations.
This classification is not carved in stone and some methods can have a qualitative or quantitative character depending on what serves the research goal better. For instance, card sorting and desirability studies can come in both quantitative (e.g. when we want to uncover a common pattern or user’s mental model) and qualitative (e.g. when we want to discover the reasons behind the patterns) versions.
Web analytics tools
Web analytics tools (eg. Google Analytics) can give us a quick overview of how users behave and navigate through our product. It’s a valuable source of data for such key metrics as bounce rate, conversion, monthly unique visitors, etc. It also provides essential demographic data, such as age, gender, and location, which enables tracking and comparing various values in different segments. Besides, web analytics tools offer an option to build conversion funnels which helps visualize the steps that users need to take in order to achieve a certain task. Thus, we can figure out what percentage of users reached the final action and at what step most users leave the funnel, which gives us a basis to investigate the possible reasons further.
Surveys
A survey is a set of questions targeted to research users’ attitudes towards different aspects of a product or a product in general. Thus, surveys are not always a source of quantitative data. By adding open-ended questions to your survey, you might gather additional context and details related to the research subject (for example, an NPS score followed by an open-ended question).
Surveys can be applied in many research situations due to their flexibility. Based on the research goal you might choose between an on-page survey, exit survey, email survey, etc. They can also be merged with other methods, such as usability testing.
Card sorting
Card sorting is a quantitative UX method used to build information architecture based on the users’ mental model. In this type of research, the participants are asked to organize individual labels into logical groups based on their own reasoning. This method allows us to identify patterns in users’ thinking which enables us to structure our product, so that the users can intuitively navigate through it.
Desirability study
A desirability study is a research method that helps us discover users’ potential attitudes and emotional responses to the product. In most cases, the user is presented with a few design directions and is asked to assign different descriptive adjectives to each of them. This way we can get an overview of how our users perceive each of these directions which might give us a hint on what alternative we should pick and pursue.
Tree testing
Tree testing is a usability method used to evaluate the hierarchical structure of a website. It’s also known as reverse card sorting. The participants receive a set of tasks aimed at finding specific information. This quantitative UX research method can help us validate how easily the users can find particular information on the website and identify where they often get stuck.
A/B testing
A/B testing is an experiment that allows us to find out how two alternative designs perform in comparison. Basically, to conduct an A/B test you need to choose one variable that you want to experiment with, elaborate two alternatives (variant A and variant B) and build a hypothesis regarding the results you want to achieve. Then the audience should be split into two equal segments in a random manner. Next variant A is presented to one user segment and variant B to the other in order to compare how well either of them performs based on the research hypothesis.
Heatmap
A heatmap is a visualized overview of user activity on a page presented with the help of colors. Basically, it shows the most popular (hot) and unpopular spots on a webpage using a range of colors from red to blue.
There are a few types of heatmaps, including click maps, mouse tracking heatmaps and scroll heatmaps. Each type results in different data about user activity (clicks, hovers, scroll, etc.) which thus helps answer different research questions:
- Do the users pay attention to the key element?
- Do the users get distracted by any other elements?
- Do the users reach certain content? etc.
Quantitative usability testing
Quantitative usability testing is a research method where researchers collect different metrics while users perform certain tasks. This type of usability testing is very similar to the qualitative one, but in case of quantitative usability testing the main focus is on specific metrics (such as time spent on task, success rate, etc.), while in qualitative usability testing we emphasize observation and getting a better understanding of particular issues.
Advantages and disadvantages of quantitative UX research
There’s no such thing as a good or bad UX research method.
The method can either be well-suited or inappropriate to your research goal. Each research method has certain possibilities and limitations which should be taken into account while making a choice. And quantitative UX research is no exception.
What can we get from quantitative UX research?
- Statistically accurate results. Quantitative UX research is intended to analyze users’ behavior en masse which ensures that the research results are more reliable.
- Fairly quick validation. As a rule, conducting quantitative UX research is less time-consuming than most qualitative UX research methods.
- Focus on specifics. Quantitative UX research is a great option when you need to narrow your focus down to certain specific details or particular aspects.
What can’t quantitative research give us?
- Precise results interpretation. Quantitative UX research on its own doesn’t give an option to follow up with the users and understand the reasons behind their behavior.
- Guarantee of unbiased outcomes. Statistics are easy to manipulate, even inadvertently. There’s a chance you might misinterpret the numbers or interpret them in favor of your own beliefs.
- Ability to emphasize with the users. In the large majority of cases, quantitative doesn’t entail getting in touch with the actual users, so it’s sometimes easy to forget that there are real people behind the statistical figures.
If you think about it, the weaknesses of quantitative UX research can be easily compensated by the strengths of qualitative UX research and the other way around. That is why we recommend a combination of these two research methods.
Quantitative UX research: best practices
1. Set a clear research goal and objectives
The first step before starting any research is setting a clear research goal and defining the objectives that will help achieve that research goal. In other words, you should decide what you want to measure so that you understand what questions you want to be answered with the help of this research. Although it might sound easy, it’s a crucial step, since goals and objectives predefine research methods and structure.
Not having a clear goal in mind might lead to a situation where your research findings don’t answer the relevant questions, which in turn means wasted time and budget.
2. Combine quantitative and qualitative data
Numerical data alone can often be hard to interpret and sometimes even misleading. On the other hand, qualitative data on its own might lack statistical significance. That’s why it’s always better to use these two data types in combination.
Imagine you’re running an on-site Net Promoter Score. If you just ask users for a numeric rating, at the end you’ll be left with a bunch of numbers which might look quite abstract on their own and which are difficult to interpret without additional context and comparison to other metrics. By simply adding a follow-up question to your survey, such as What’s the main reason for your rating?, you can gain much more value. In combination with qualitative data, the numbers become more meaningful and you get insights into what the users appreciate about your product and what they struggle with.
The same applies to the data collected from web analytics tools, such as Google Analytics. You might notice some pattern in your quantitative data, e.g. your bounce rate has become higher or a significant number of users leave your website on a specific page. The causes for such tendencies might be various and identifying the true reason is practically impossible without observing the users or conducting a series of in-depth interviews.
Although quantitative data might serve as a great incentive or an indicator of a problem, in most cases we have to back it up with qualitative details in order to find out what the actual problem is and how we can possibly solve it.
It also works the other way around. For example, imagine that after having conducted a series of usability tests, you found out that most of the participants admitted having difficulty filling out a form in your application. In this case, you might want to resort to Google Analytics to compare these findings with some large-scale data. Using funnels you can, for instance, check what percentage of users submit the form after starting it. You can also find out at what step the users exit the process. This way you can obtain more reliable and validated findings.
3. Choose an adequate sample size and target group
Quantitative UX research implies that in order to obtain statistically significant data, research should be conducted on a larger sample size. But how many participants is enough? More participants doesn’t necessarily mean better results, but will likely mean more expense. So, where is the happy medium between wise budget management and precise research results?
The number may vary depending on the research method and your tolerance to measurement errors (there will always be some imprecision, but in some cases a higher margin of error might be more acceptable than in others). According to Nielsen Norman Group, more often than not a sample size of 40 participants is satisfactory for quantitative research methods (source).
Besides, depending on your research goal you might want to target different segments of your audience based on age, location, and other demographics.
4. Avoid ambiguity in research questions
It’s especially important in the case of quantitative UX research to make sure your questions are as unambiguous as possible. Otherwise, we’re running the risk of disturbing and/or biasing the test results.
Let’s have a look at an example survey question.
On a scale from 1 to 10, how satisfied are you with our service and customer support?
This question is flawed for two reasons:
- Strictly speaking, this question contains two separate questions - about the service in general and the customer support. It might corrupt the results because a user might want to rate these two aspects differently.
- By including the word “satisfied” in the question we bias the users into thinking they are satisfied which might not be the case.
A good approach here would be to divide the question into two and reframe in a more neutral tone: On a scale from 1 to 10, how would you rate our service? and On a scale from 1 to 10, how would you rate our customer support?
5. Don’t modify the research structure on the go
In qualitative UX research, it’s permissible to modify the research structure and conditions from session to session (e.g. changing the form of the task or removing it altogether if you noticed after a few interviews that the task doesn’t provide you with the information you expected to get, or confuses the users). It might make it more difficult to average the overall results, but at the end of the day in qualitative research the goal is to get insights, not statistically perfect data.
In the case of quantitative UX research, the situation is different. The study structure should stay the same throughout the research in order to ensure statistically precise results. Thus, it’s crucial to make sure that your research tasks and questions are relevant to your research goal beforehand.
6. Beware of confirmation bias
Since numerical data in most cases requires additional interpretation, it’s easier to yield to confirmation bias (the tendency to interpret information so that it supports one’s beliefs and assumptions). It is very easy to succumb to the impulse to favor and highlight the information that proves your point of view and ignore the facts that demonstrate the opposite.
What can be done to prevent our biases from disturbing the research results?
- Write down all the assumptions that you have in your head before beginning the research. It’s even better if you could do this together with your team. Ask the participants to jot down all their thoughts and assumptions about the outcome of the upcoming research. Once you get the assumptions out of your head and see them written down on paper, it’s easier to stay aware of them and avoid being biased.
- Combine and compare multiple data points. If you collect data from different channels, it would be more difficult to yield to one-sided interpretation.
- Challenge your ideas and hypotheses consistently. Although it might feel unnatural, embrace disagreement. The best way to defeat confirmation bias is adopting the mindset of constantly searching for other perspectives.
7. Look at data in context
Stand-alone quantitative data is rarely able to show us the full picture. Taken out of the context it can be extremely misleading. That’s why it’s important to combine a few research methods in order to get the fullest overview of the situation and interpret the data correctly.
Let’s have a look at a few context-related examples:
The overall Net Promoter Score of the product in October is 0, the result in November is also 0. What can we conclude from this data alone? It looks like nothing much has changed, in both months we have an equal proportion of promoters and detractors. However, if we dig a little deeper and look at the ratings given to us in October and November by our detractors, it might turn out that in October most of the detractors gave us a very low score (0-1), and in November detractors were giving us ratings from 4 to 6. This doesn’t change the overall score, but still demonstrates the dynamics.
Another possible example is related to scroll heatmaps. Let’s imagine you look at the heatmap of your website’s homepage and see that a great percentage of users have scrolled the page almost to the very bottom. Is it a good sign or a bad sign? Actually, in many situations, it might be considered as something good. If users scroll down, they find the content on the page interesting and engaging, right? Well, it depends. It might also mean that they are not able to find what they are looking for or don’t understand how to navigate the website. So, in order to understand the actual situation, you might need to take a look at some other metrics (such as bounce rate, conversion, etc.) or possibly even employ some additional research methods (usability testing, observations through Hotjar recordings, user interviews and so on).
Is it worth using quantitative UX research in product development?
In short: YES! We always encourage our partners to run as much research as needed. They provide the scrum team with an amazing amount of knowledge that should be used during the digital product development process. Products created around the user’s needs and behaviors always offer much more business quality than those created around the needs of stakeholders or investors.
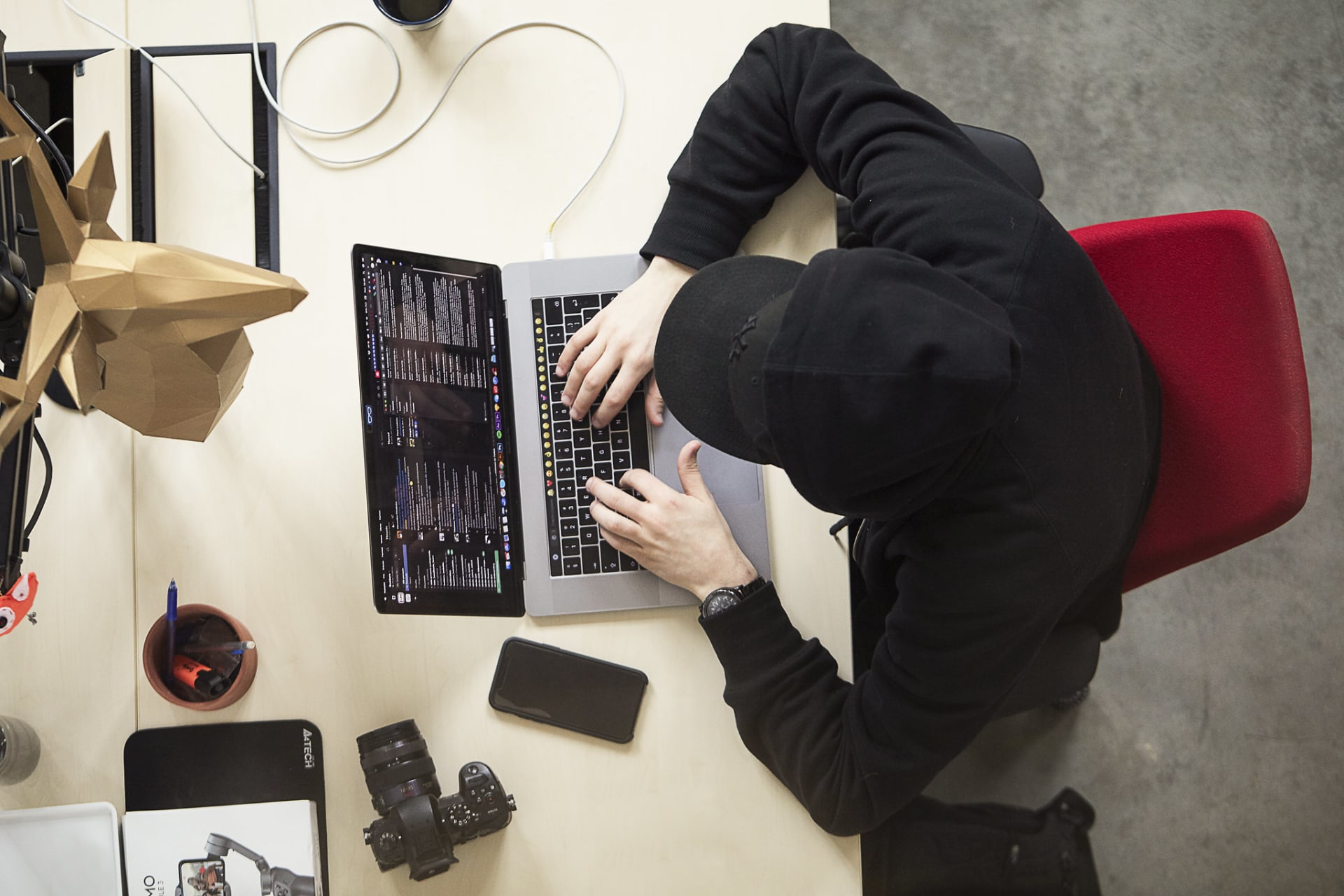
Share this article: